The primary focus of my research is in ecological statistics. In particular, I have worked with the U.S. Forest Inventory and Analysis (FIA) Program to analyze different types of forestry data.
Spring-Summer 2019
The National Forest Inventory and Analysis (FIA) Program of the US Forest Service produces a yearly inventory of national forest attribute estimates like above-ground biomass. The FIA uses a statistical technique called post-stratification to improve the variance of these estimates. Post stratification involves weighting the attribute estimates based on geographic auxiliary data.
The Interior West unit of the FIA is currently using a forest probability map for post-stratification. This scheme has only two strata, 'forest' (corresponding to high forest probability) and 'nonforest'. Over the course of this research, we investigated several alternative post-stratification schemes that lower the variance of forest attribute estimates when compared with the current scheme. The best candidate scheme was also based on the forest probability map, but with four strata instead of two. We designed these strata to more accurately reflect Interior West geography, with multiple strata corresponding to low forest probability instead of just one. This proposed scheme consistently reduces the variance of forest attribute estimates compared to the current sceme.
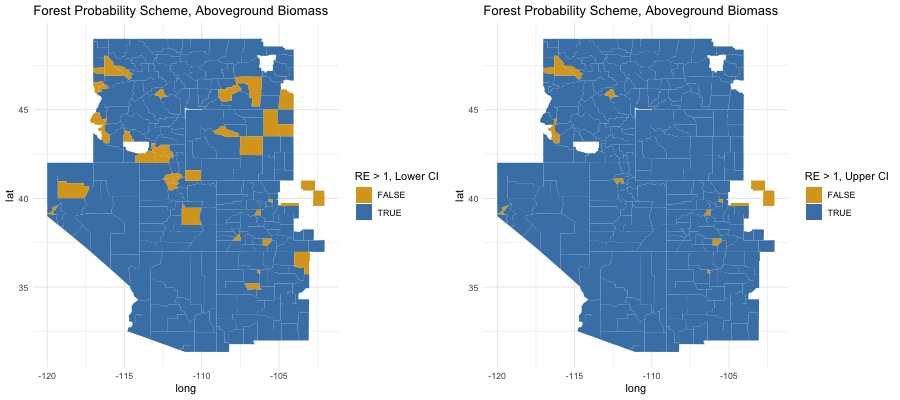
This project was published in an FIA Technical Report (page 268) and is freely available.
Fall 2020 - Spring 2021
Forest biomass is a difficult and time-consuming quantity to measure. In order to get biomass estimates at a large scale, the FIA uses biomass data from destructively sampled trees to find relationships between easily measured quantities (like tree diameter) and biomass. However, the process of measuring the biomass of a felled tree is complex, and it is not precise. This uncertainty is then carried through to larger-scale biomass estimations.
In this work, we seek to quantify the uncertainty in the process of deriving a measure of biomass from a sampled tree. If we can find a reliable estimate of this uncertainty, then we can propogate it through the process of estimating biomass on county-level or state-level forests, leading to a measure of variability in large-scale forest estimation. We are working with Northern Arizona University (NAU), whose field crews destructively sampled several poderosa pine trees in northern Arizona, providing us with the data needed to complete this project.